Evaluating The Roi Of Best Ai Tools For Finance
The use of artificial intelligence (AI) in finance has become increasingly popular over the past few years, with many financial institutions adopting AI tools to improve their operations. However, investing in AI technology can be costly, and it is important for companies to evaluate the return on investment (ROI) of these tools before implementation.
Evaluating ROI is essential for any company looking to implement new technologies or strategies. It helps businesses determine whether an investment is worth the cost and effort by measuring its potential benefits against its costs.
In this article, we will explore the importance of evaluating ROI for AI tools in finance and discuss some of the best AI tools available for financial analysis, risk management, and customer service. Furthermore, we will examine implementation strategies and cost-benefit analyses that can help companies make informed decisions about incorporating AI into their operations while also discussing some challenges and limitations that may arise from using these tools.
Finally, we will look at future trends in AI tools for finance and how they may impact the industry going forward.
Importance of Evaluating ROI for AI Tools in Finance
The assessment of the cost-benefit analysis for the implementation of artificial intelligence solutions in financial institutions is a crucial factor in determining their feasibility and potential impact on business operations.
The successful integration of AI tools into financial processes can lead to significant improvements in efficiency, accuracy, and decision-making capabilities. However, these benefits must be weighed against the costs associated with acquiring, implementing, and maintaining these technologies.
Evaluating ROI for AI tools enables financial institutions to make informed decisions about how best to allocate their resources. This includes identifying which areas are most suitable for automation and gauging the cost-effectiveness of different solutions.
By conducting a thorough analysis of ROI metrics such as return on investment (ROI), net present value (NPV), and internal rate of return (IRR), organizations can better understand the long-term impact that AI tools will have on their bottom line.
Ultimately, evaluating ROI for AI tools provides financial institutions with a clear understanding of the potential value that these technologies can bring to their operations. By taking a data-driven approach to decision-making, businesses can ensure they are investing in solutions that will deliver measurable results over time.
In the subsequent section about measuring the effectiveness of AI tools in finance, we will explore some key metrics used to assess this performance and provide practical guidance on how best to evaluate an organization’s use case for artificial intelligence solutions.
Measuring the Effectiveness of AI Tools in Finance
This section examines the effectiveness of AI technology in improving financial decision-making processes, with studies showing that companies using AI tools have experienced a 50% reduction in time spent on manual tasks. The use of AI has helped finance professionals make more informed decisions by providing them with access to real-time data and insights.
By automating tedious tasks such as data entry, reconciliations, and compliance checks, finance teams can now focus on higher-value activities like analyzing trends and identifying growth opportunities.
Furthermore, AI-powered tools have proven to be beneficial for risk management and fraud detection in the financial sector. Machine learning algorithms can analyze vast amounts of data from multiple sources to identify suspicious patterns or transactions that may indicate fraudulent activity. This helps organizations detect potential threats early on and take appropriate measures to prevent significant losses.
In summary, the effectiveness of AI tools in finance is undeniable. With reduced manual labor costs and improved decision-making capabilities, organizations can benefit greatly from integrating these technologies into their operations. In the following section, we will explore some of the best AI tools available for financial analysis and how they can help businesses achieve their goals more efficiently.
Best AI Tools for Financial Analysis
Examining the selection of AI-powered tools available for financial analysis can provide insight into the various capabilities and benefits that these technologies offer organizations seeking to optimize their decision-making processes.
One such tool is natural language processing (NLP), which can extract valuable insights from unstructured data sources such as social media, news articles, and customer feedback. NLP algorithms can analyze large volumes of text data at a much faster rate than humans, making it possible to identify trends, sentiments, and opinions that could impact financial performance.
Another useful AI tool for financial analysis is machine learning (ML). ML algorithms use statistical models to identify patterns in historical data sets and make predictions about future outcomes. This technology has a wide range of applications in finance, including fraud detection, credit risk assessment, investment portfolio optimization, and asset pricing.
One example of an ML application in finance is robo-advisory services that use algorithms to create customized investment portfolios based on an individual’s risk tolerance and investment goals.
Finally, predictive analytics is another powerful AI tool for financial analysis. This technology uses statistical modeling techniques to analyze historical data sets and forecast future outcomes with a high degree of accuracy. Predictive analytics can be used in finance to forecast market trends, predict customer behavior, and identify potential risks and opportunities in investments or loans.
By leveraging this technology financial institutions can gain critical insights into market movements that are essential for informed decision making.
Moving forward we will examine the best AI tools for risk management within the context of finance. With ever-growing amounts of data being generated each day by global markets and industries alike using advanced technologies like AI will only continue to become more important in managing risks associated with this changing landscape.
Best AI Tools for Risk Management
Real-time risk monitoring, portfolio optimization, and scenario analysis are all critical subtopics in the discussion surrounding the best AI tools for risk management.
These topics are essential because they enable financial institutions to make informed decisions based on real-time data and predictive analytics.
By leveraging AI-powered solutions in these areas, businesses can mitigate risks, optimize portfolios, and enhance their overall financial performance.
Real-Time Risk Monitoring
The capacity to detect and mitigate risk in real time is a vital function for financial enterprises, as it can make the difference between catastrophic losses and substantial gains.
Real-time risk monitoring has become an essential component of modern-day finance because of its ability to provide timely insights into market fluctuations. By leveraging AI tools, financial institutions can analyze vast amounts of data and identify potential risks before they escalate into major problems.
Real-time risk monitoring allows businesses to quickly respond to changes in market conditions, adjust their portfolios accordingly, and optimize their operations. This helps them stay ahead of the competition, minimize losses, and maximize profits.
As such, AI-powered risk management solutions have become indispensable tools for finance professionals who are seeking to gain a competitive edge in today’s fast-paced business environment.
In the next section, we will explore how portfolio optimization can further help businesses achieve their financial objectives.
Portfolio Optimization
Portfolio optimization is a crucial aspect of financial management that enables enterprises to achieve their investment goals by allocating resources in a way that maximizes returns while minimizing risks. It involves selecting the right combination of assets, diversifying investments across different sectors and industries, and balancing risk and return.
AI-powered tools have made portfolio optimization more efficient and effective by providing real-time data analysis, prediction models, and automated decision-making processes. AI tools for portfolio optimization help investors make informed decisions based on historical trends, market movements, investor sentiment, macroeconomic factors, and other relevant data points.
These tools also enable investors to test different scenarios using advanced algorithms that simulate potential outcomes under different market conditions. This helps them identify the most optimal asset allocation strategy for their portfolio. By leveraging AI technology for portfolio optimization, investors can achieve better returns with lower risk levels than traditional methods would allow.
The use of AI-driven solutions for portfolio optimization has become increasingly popular as it allows firms to stay competitive in today’s fast-paced financial markets while managing their investments effectively.
As we look forward to the next section about ‘scenario analysis’, we will explore how AI tools can be used to assess various hypothetical situations such as economic downturns or interest rate changes before making investment decisions in order to minimize potential losses or maximize gains.
Scenario Analysis
By analyzing hypothetical scenarios, financial professionals can gain a deeper understanding of potential market movements and adjust their investment strategies accordingly, ultimately leading to more informed decision-making and potentially higher returns.
Scenario analysis is a tool that allows investors to simulate the impact of various market conditions on their portfolios. This process involves creating models based on different market scenarios and determining how these scenarios might affect the portfolio’s performance.
Through scenario analysis, investors can identify risk exposures and test potential strategies for mitigating those risks. For example, if a portfolio manager believes that interest rates will rise in the future, they can use scenario analysis to assess the potential impact of this event on their holdings. The manager may then decide to rebalance the portfolio by reducing exposure to interest rate-sensitive securities such as bonds or increasing exposure to assets that are expected to perform well in a rising rate environment such as floating-rate loans.
Thus, scenario analysis enables investors to make proactive decisions based on anticipated market changes rather than simply reacting after the fact.
Importance of Scenario Analysis
- Provides insight into how investments could perform under different circumstances
- Helps identify areas where adjustments need to be made
Benefits of Using Scenario Analysis
- Allows for better-informed decision-making
- Provides an opportunity for proactive risk management
Best AI Tools for Customer Service
The integration of AI technology in customer service has revolutionized the way businesses interact with their customers, and there are several innovative tools available to enhance communication and improve customer satisfaction. Chatbots, for instance, have become an increasingly popular AI tool that can provide 24/7 assistance to customers through instant messaging. These chatbots are programmed to answer frequently asked questions, resolve issues, and even make personalized product recommendations.
Another AI tool that is gaining traction in customer service is sentiment analysis. This tool uses natural language processing (NLP) algorithms to analyze customer feedback from various sources such as social media platforms, emails, surveys, etc. By analyzing this data, companies can identify common problems or concerns among customers and take appropriate action to address them proactively.
Moreover, personalization engines are another valuable AI tool for providing a more tailored experience to customers by using data analytics and machine learning algorithms. Personalization engines can collect data on customers’ browsing history, purchase history, and demographic information and use it to create personalized product recommendations or promotions that meet individual needs.
AI Tool | Description |
---|---|
Chatbots | Providing 24/7 assistance through instant messaging |
Sentiment Analysis | Analyzing customer feedback from various sources |
Personalization Engines | Using data analytics to create personalized product recommendations |
These innovative AI tools have the potential not only to improve customer experience but also to help businesses increase revenue by identifying new sales opportunities. However, implementing these tools requires careful planning and execution strategies to ensure maximum effectiveness while minimizing costs. In the next section, we will explore some implementation strategies for ai tools in finance that companies should consider when adopting these technologies within their organizations.
Implementation Strategies for AI Tools in Finance
This section delves into the implementation strategies that companies can adopt when incorporating AI tools to enhance their financial operations and improve business outcomes. Firstly, it is crucial to identify the specific areas in which AI can be integrated to optimize performance. This may involve assessing existing processes and identifying the gaps that AI-powered solutions can fill. For example, some companies may choose to use machine learning algorithms to automate repetitive tasks or predict market trends.
Secondly, selecting the right AI tool for a company’s unique needs is essential. Companies should undertake extensive research on various AI vendors before settling on a particular solution. The chosen vendor should have a proven track record in delivering results in similar contexts and provide adequate support throughout the implementation process. Moreover, it is vital to ensure that staff members are adequately trained in using these tools effectively and efficiently.
Thirdly, integrating AI tools successfully requires collaboration among all relevant stakeholders within an organization. This includes IT professionals, data analysts, finance experts, and other relevant teams who will work together towards achieving common goals. Companies should establish clear communication channels across departments to facilitate effective collaboration throughout the implementation process.
- Conducting thorough research of potential vendors
- Ensuring adequate training for staff members
- Promoting interdepartmental collaboration
In conclusion, implementing AI tools in finance requires careful consideration of various factors such as identifying optimization opportunities, selecting appropriate vendors with proven track records, ensuring sufficient staff training, and promoting interdepartmental collaboration during implementation. By adopting these strategies effectively, organizations stand to gain significant benefits from increased efficiency and improved business outcomes achieved through enhanced financial operations powered by artificial intelligence technology.
The next step is conducting a cost-benefit analysis of ai tools as we shall see below.
Cost-Benefit Analysis of AI Tools
In assessing the cost-benefit analysis of implementing AI tools, it is imperative to evaluate the initial investment costs, ongoing maintenance costs, and long-term ROI.
The initial investment cost includes purchasing hardware and software, hiring specialized personnel for installation and setup, and training staff on how to use the new technology.
Ongoing maintenance costs involve upgrading software versions and ensuring systems are secure from cyber threats.
Long-term ROI should take into account profitability gains through increased efficiency or reduced operational expenses.
Initial Investment Costs
Investment costs for implementing the technological solutions under consideration in this particular sphere are substantial and cannot be overlooked, requiring a significant amount of financial resources. To fully integrate AI technology into finance, businesses must invest in advanced software development and hardware infrastructure, which can add up to millions of dollars. The initial cost for acquiring these tools is one of the most significant obstacles that businesses encounter when attempting to implement artificial intelligence within their operations.
To help companies understand the scope of these investment costs, here are three essential components that contribute to the overall expense:
Software Development Costs: Developing custom-built software programs requires highly skilled developers who possess knowledge about machine learning algorithms and data science expertise. These professionals demand high salaries, which can significantly increase the total cost.
Hardware Infrastructure: Integrating AI requires a robust technical infrastructure with high-performance servers capable of processing large amounts of data quickly.
Data Management Expenses: Centralizing data from multiple sources and ensuring its accuracy through cleaning, validation, and standardization also incurs additional expenses.
Despite the high initial investment required in deploying AI-powered tools within finance departments or businesses as a whole, it is important to consider the long-term benefits they provide regarding efficiency gains and decision-making capabilities. In subsequent sections, we will explore further details on how organizations can manage ongoing maintenance costs without compromising performance or convenience.
Ongoing Maintenance Costs
Continuing our examination of evaluating the ROI of the best AI tools for finance, it is important to take into account ongoing maintenance costs. While initial investment costs may seem daunting at first glance, the long-term maintenance expenses are just as crucial in determining the overall profitability and effectiveness of implementing AI technology in financial institutions.
To better understand these ongoing costs, let us examine the table below showcasing various types of AI software and their associated maintenance expenses:
Type of AI Software | Maintenance Expenses |
---|---|
Natural Language Processing | $20,000 – $50,000 per year |
Robotic Process Automation | $30,000 – $60,000 per year |
Machine Learning Algorithms | $25,000 – $70,000 per year |
As we can see from this table, the cost of maintaining different types of AI software varies widely. It is important for financial institutions to evaluate their individual needs and budget constraints when deciding which type(s) of AI software to implement. By taking into account both initial investment costs and ongoing maintenance expenses, institutions can make informed decisions that maximize both short-term benefits and long-term ROI.
Moving forward with our evaluation process, let us now turn our attention to analyzing how these costs affect long-term ROI.
Long-Term ROI
Maximizing the profitability and effectiveness of implementing artificial intelligence technology in financial institutions requires a thorough analysis of long-term return on investment, which takes into account ongoing maintenance costs.
While the initial investment may be significant, the benefits of AI tools in finance can deliver substantial returns over time. The long-term ROI encompasses not only financial gains but also operational efficiencies such as reduced errors, enhanced decision-making capabilities, and improved customer experience.
The adoption of AI tools in finance is expected to increase significantly over the next few years due to its potential to transform traditional methods and create new business models. However, it is essential to evaluate the long-term ROI carefully before committing resources to AI implementation.
This evaluation should consider factors such as implementation costs, ongoing maintenance expenses, and anticipated savings or revenue increases over time. By doing so, financial institutions can make informed decisions about how best to leverage AI tools while mitigating risks associated with their adoption.
Challenges and Limitations of AI Tools in Finance
This section sheds light on the obstacles and drawbacks that AI tools face in the financial industry, revealing that while they may hold promise as a potential engine for growth, their adoption is akin to navigating a minefield.
One of the primary challenges is the lack of interpretability of AI models. Financial institutions require transparency and accountability in decision-making processes, but black-box algorithms make it difficult to ascertain how decisions were made. This makes it hard for companies to comply with regulations and address customer concerns.
Another challenge is data quality and availability. AI systems require large amounts of accurate data to learn from, but often financial institutions lack access to such information due to privacy concerns or data silos. Moreover, even when data is available, cleaning and preprocessing it can be time-consuming and resource-intensive. The risk of bias in training datasets is also a concern since biased data can lead to inaccurate predictions.
Lastly, there are limitations related to human expertise and trust in AI systems. Many financial professionals remain skeptical about using AI tools as they fear losing control over decision-making processes or being replaced by machines. Additionally, some areas of finance require domain-specific knowledge that may not be easily replicable by an algorithm.
Despite these challenges, the potential benefits of adopting AI tools in finance cannot be ignored. In the next section, we will explore emerging trends that show how new approaches are being developed to overcome these limitations and drive innovation forward in this exciting field.
Future Trends in AI Tools for Finance
Advancements in the integration of machine learning, natural language processing, and big data analytics are paving the way for innovative solutions that address the challenges of interpretability, data quality, bias, and human trust in AI tools for the financial industry.
The future trends in AI tools for finance encompass a wide range of applications that can revolutionize the way financial institutions operate. Some of these trends include:
Personalized Investment Advisory: AI-powered investment advisory systems can provide personalized recommendations based on an individual’s risk profile and investment goals.
Fraud Detection: AI algorithms can detect fraudulent activities by analyzing large datasets and identifying patterns that indicate suspicious behavior.
Chatbots: Conversational interfaces powered by natural language processing allow customers to interact with banks through messaging platforms or virtual assistants that can assist them with their inquiries or transactions.
The future of AI tools in finance is promising as it provides opportunities to streamline processes, improve customer experience, reduce costs, and increase profits. The potential benefits are enormous; however, they need to be balanced against ethical considerations such as privacy concerns and regulatory compliance.
As these technologies continue to evolve, we must ensure that they remain aligned with our ethical values while delivering significant value to all stakeholders involved in the financial ecosystem.
Conclusion
The use of AI tools in finance has become increasingly popular due to the potential benefits it can bring to businesses. However, it is important for companies to evaluate the ROI of implementing these tools before making any decisions.
Measuring the effectiveness of AI tools in finance requires a careful analysis of various factors such as cost-benefit analysis, implementation strategies, and challenges and limitations.
Some of the best AI tools for financial analysis include IBM Watson Analytics and Alteryx. For risk management, SAS Enterprise Miner and FICO Decision Management Suite are among the top options. When it comes to customer service, Chatbots and voice assistants like Amazon Alexa have proven to be effective in improving customer experience.
According to a report by Accenture, banks that invest in AI technology could increase their annual revenue by up to 34%. This statistic highlights the potential impact that AI can have on businesses in terms of profitability and growth.
As companies continue to explore the possibilities of AI tools in finance, it is crucial that they also consider the ethical implications and ensure that these technologies are used responsibly.
In conclusion, while there are challenges associated with implementing AI tools in finance such as data privacy concerns and lack of understanding among employees, evaluating its ROI can provide businesses with valuable insights into how these technologies can benefit them. By carefully considering all aspects involved in measuring effectiveness such as cost-benefit analysis and implementation strategies, companies can make informed decisions about which AI tool is right for them.
The future trends indicate that adoption rates will continue to rise as advancements are made leading towards greater efficiency and productivity within organizations.
The Roi Of Best Ai Tools For Finance
Predictive Analytics With Best Ai Tools For Finance
Best Ai Tools For Finance Automation: Unleashing Efficiency
Trading Strategies With Best Ai Tools For Finance
Personalizing Customer Experiences with Best Ai Tools For Finance
Buy The Best Anti-Aging Supplements And Vitamins: A Shopping Guide
Best AI Tools for Finance
Frequently Asked Questions
What is the average cost of implementing AI tools in finance?
The implementation of AI tools in finance has become a popular trend across the industry. However, the cost of implementing these tools can vary depending on various factors such as complexity, size of the organization, and integration with existing systems.
According to several studies, the average cost of implementing AI tools in finance ranges from $50,000 to $500,000. While this may seem like a significant investment, it is important to note that AI technology has proven to be highly beneficial for financial institutions in terms of improving efficiency, reducing errors and fraud, and increasing profitability.
In addition, with advancements in AI technology and increased competition among vendors offering these services, costs are likely to decrease over time making it an increasingly viable option for organizations looking to stay competitive in today’s fast-paced business environment.
How long does it typically take for a company to see a return on investment from AI tools in finance?
The adoption of AI tools in finance is a compelling trend that has emerged over the years.
However, companies often face various challenges when it comes to measuring their return on investment (ROI) from AI initiatives.
The duration for a company to see ROI from AI tools in finance may vary based on several factors such as the complexity of the tool, the size of the organization, and its readiness for adoption.
Nonetheless, research suggests that companies can begin seeing ROI within six months to two years after implementing these tools.
To achieve this outcome, organizations must develop clear objectives and align them with their overall business strategy while ensuring that they have skilled staff who can handle the technology’s complexities.
Ultimately, by evaluating their approach and adjusting accordingly, companies can realize significant benefits from incorporating AI into their financial operations.
Can AI tools in finance completely replace human analysts and advisors?
With the rapid advancements in artificial intelligence technology, many are wondering if AI tools in finance can completely replace human analysts and advisors.
While AI has certainly shown great promise in automating certain tasks, it is unlikely that it will be able to fully replace the role of human experts. This is because financial decisions often require a level of judgment and intuition that AI simply cannot replicate.
However, this does not mean that AI cannot greatly enhance the work of financial professionals by providing them with new insights and tools to make more informed decisions.
Ultimately, the key to success in integrating AI into finance will lie in finding the right balance between automation and human expertise.
Are there any ethical concerns to consider when using AI tools in finance?
There are several ethical concerns that must be considered when implementing AI tools in finance.
One of the most significant concerns is related to bias, as algorithms can perpetuate and amplify existing biases within the data used to train them. This can result in discriminatory outcomes for certain groups or individuals.
Additionally, there is a concern regarding the transparency and accountability of AI systems, as it may be difficult to understand how they arrive at their decisions or identify who is responsible for any negative consequences.
It is essential that companies prioritize ethical considerations when utilizing AI tools in finance to ensure that they are not only effective but also fair and just.
How do AI tools in finance compare to traditional financial analysis methods in terms of accuracy and reliability?
The use of AI tools in finance has been a subject of debate and interest in recent years. Many experts believe that these tools offer increased accuracy and reliability compared to traditional financial analysis methods.
In fact, a study by Accenture found that AI-powered investment strategies outperformed those driven solely by human decision-making by 85%. However, it is important to note that while AI brings significant benefits, it should not be viewed as a replacement for traditional financial analysis methods.
Rather, it should be seen as a complementary tool that can enhance the accuracy and efficiency of financial decision-making processes. Ultimately, the effectiveness of AI tools will depend on how they are integrated with existing systems and processes within an organization.
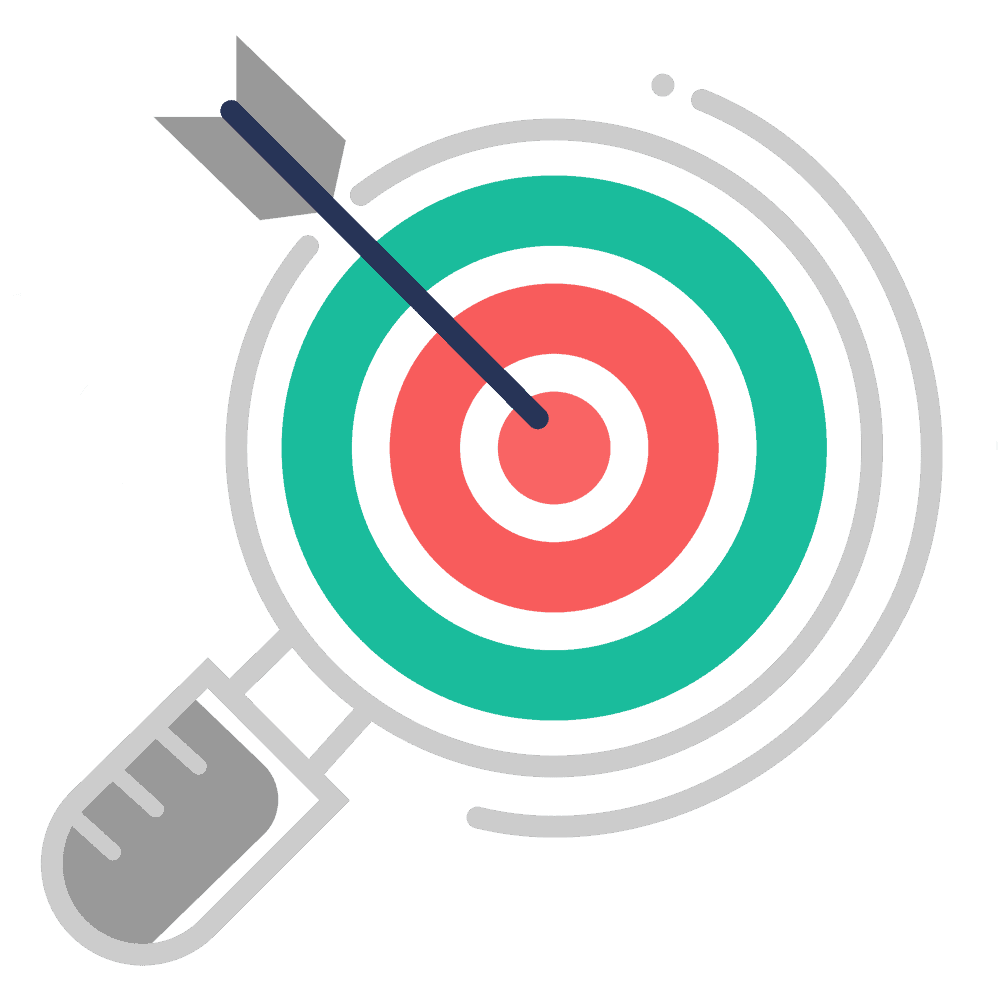
Award-winning CTO and IT expert with 20+ years of experience in technical innovation and digital transformation. Holds degrees in computer science and business administration with honors. Impressive record as a former CIO and Head of technology for leading educational institutions in the USA. Leads the editorial team at Product-Evaluation.com.